Sep 26, 2024
AI in Industry 4.0: More manual labor than expected
The use of artificial intelligence (AI) in manufacturing is often hailed as a revolutionary step toward greater efficiency and automation. However, for many companies, the journey still involves a lot of change. Far from being plug-and-play, implementing AI in a manufacturing environment requires a surprising amount of manual work and human expertise. But it is worth the effort.
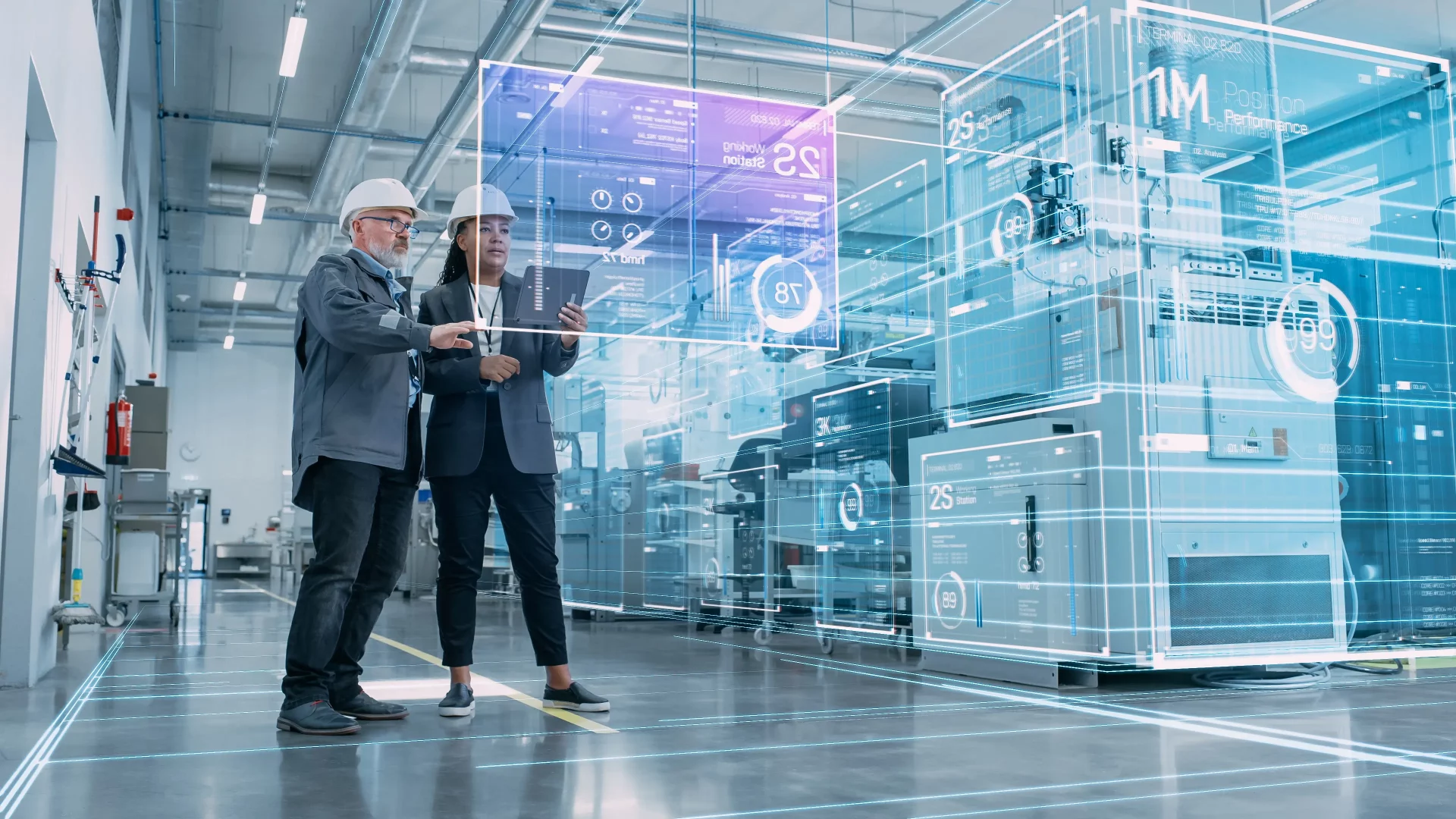
Currently, easy access to generative, language-based AI tools like ChatGPT gives the impression that AI can be easily integrated into all business processes and deliver results immediately. This may be true for some business areas such as marketing, sales, or customer service. For example, the output might be a text or image, automated answers to frequently asked questions, lead generation automation, or product advice. Generative AI specializes in generating content that is indistinguishable from human work. It focuses on tasks that require natural language processing. But what about manufacturing, where the requirements are much more specialized and complex?
Generative vs. classical AI
Generative AI can also play an important role in manufacturing, for example in creative processes such as the design and prototyping of new products - although the models and tools used for this are still far from the quality of text generation tools. However, the main reasons for the increased use of AI in manufacturing are efficiency gains and cost reductions. Generative AI is no longer sufficient in these areas where specialized knowledge, critical decisions, or physical interactions are anticipated or required. This is due to its data sources: it has been trained on billions of global data sets, based on statistical models, from which it recognizes and derives patterns, rules and structures, and generates new ones based on them. Industrial manufacturing, on the other hand, is customized. It requires specific AI tools. Its applications and goals, the data it uses, the way it interacts, and the type of output it produces are very different from those of generative AI. Like generative AI, they are based on machine learning. They also include rule-based systems that deterministically incorporate manufacturing expertise into the algorithms. These custom systems must be not only effective, but also transparent and understandable to function properly in the complex manufacturing environment.
Transparency in learning AI
Almost all AI models in use today are based on machine learning, i.e. learning algorithms that improve themselves and learn from their experience.
The performance and effectiveness of machine learning models depends on the quality, quantity, and relevance of the data used.This data comes from a variety of sources. These include sensors on machines, systems, and production lines; data from operations, logistics, maintenance, and quality control; human input; and external sources such as weather data.
Within the models, this data is processed, analyzed, and correlated. The algorithms identify patterns, relationships and trends that are often not obvious to humans. These insights enable the models to make predictions, support decisions, or take autonomous actions.
The ability of models to continuously learn from new data makes them particularly valuable in dynamic environments such as those found in industry. Learning AI can adapt to changing conditions, incorporate new production processes, or respond to market changes.At the same time, this places high demands on data management and quality. Incorrect or biased data can lead to incorrect conclusions, so careful data selection and preprocessing are essential.
Another important aspect is the interpretability of the models. While some machine learning techniques, such as decision trees, are relatively transparent in their decision-making process, others, especially deep learning models, are often considered "black boxes". Here, the processes are not visible and the results cannot be understood neutrally. This can be a challenge in sensitive areas where traceability and explainability are important. This makes it all the more important to ensure the quality of the underlying data to ensure the reliability and accuracy of AI models.
Data quality is crucial
For many organizations, the sheer complexity and volume of data is a challenge in itself.
This is especially true when data is stored separately in silos-which is still the case in many organizations. Data silos lead to a fragmented data landscape and a significant loss of quality. It is often outdated, inconsistently formatted, and only accessible to certain departments or groups of people. When these data sets are fed into AI, the result is inconsistent data formats, duplication, gaps and conflicting information.AI decisions are then based on this fragmented information. This makes it difficult to achieve clear analysis, gain new insights and make informed decisions.
In addition, organizational structures must be adapted through change management. Moving to a data ecosystem with data consistency, transparency, and interoperability is key. Data governance establishes policies and standards, while cultural change promotes data transparency and sharing. Read more: https://ascon-systems.de/en/aim/
People set the goals
Despite technological advances, including automation, people remain the critical factor in implementing AI systems in manufacturing processes. Human expertise is critical to effectively train, monitor, and adjust AI models, which requires a deep understanding of the specific production environment and technical requirements. It is also critical in defining the requirements for the outcome that the AI should deliver by asking precise questions.
Re-evaluation plays a key role: Precisely because an AI project is constantly evolving based on currently available data, results, and new insights, it is necessary to regularly review and, if necessary, adjust the goals and values. Otherwise, in the worst case, you could end up at a different point in the process than desired.
In all of this, human critical judgment and ethical considerations are central. They ensure that AI systems are used responsibly and for the benefit of the entire organization.